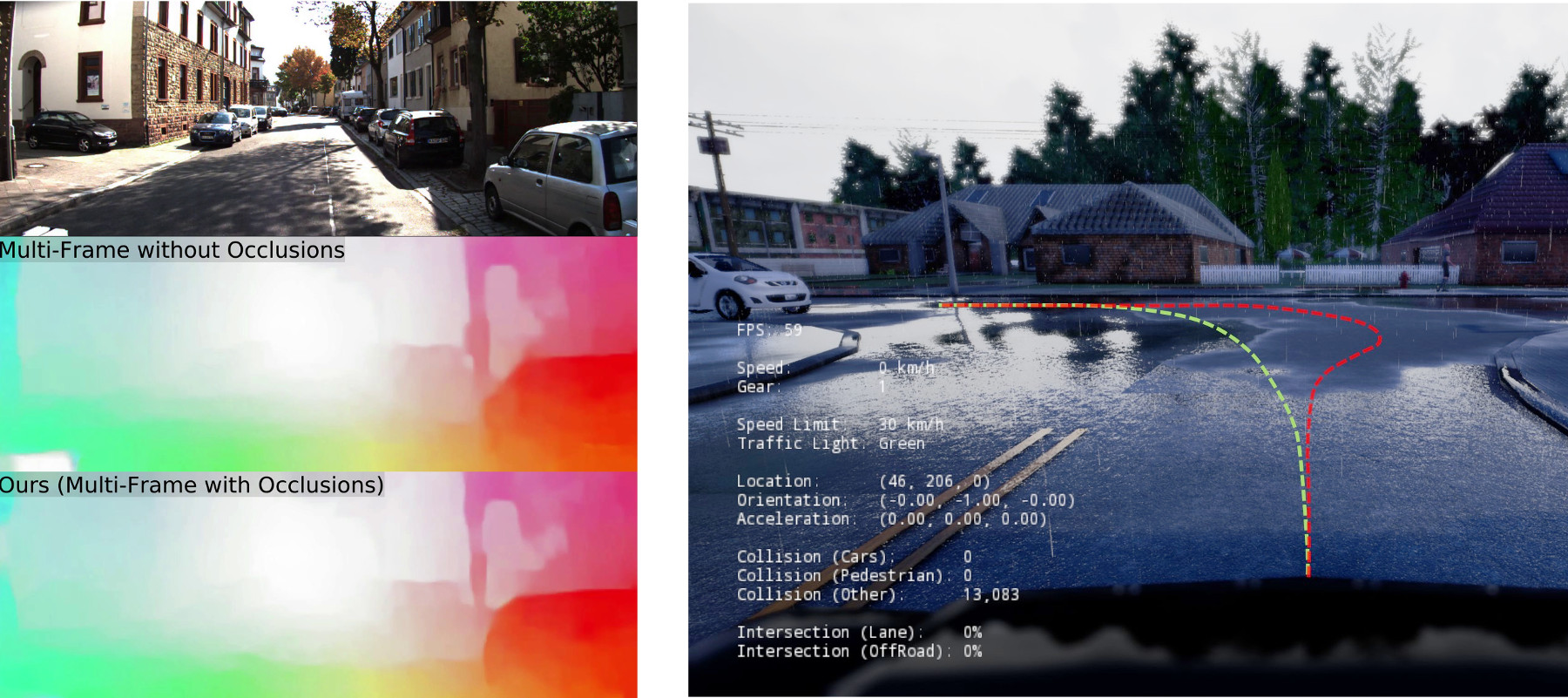
Understanding scenes in motion is crucial for intelligent systems. We have developed novel self-supervised approaches for learning optical flow and proposed a model for learning driving affordances from video sequences using limited supervision.
Intelligent systems as well as many computer vision tasks benefit from an accurate understanding of object motion as well as the interplay between scene elements. However, motion is even less constrained than geometric structure and obtaining ground truth is difficult. Furthermore, besides pure 2D image motion, systems operating in the 3D world require access to 3D motion information. Our research addresses all of these aspects and combines them for sensori-motor control tasks such as autonomous driving.
Optical flow is the problem of estimating 2D motion in the image plane. While deep learning has led to significant progress, large amounts of training data are required. We have addressed this problem by developing SlowFlow, a space-time tracking technique for generating training data using high-speed cameras. Moreover, we have proposed a new model for self-supervised flow estimation from multiple frames, explicitly accounting for occlusions.
To recover 3D motion, we have developed a state-of-the-art 3D scene flow estimation technique which exploits recognition (bounding boxes, instance segmentation and object coordinates) to support the challenging matching task. Furthermore, with SphereNet, we have investigated how optical flow can be extracted from spherical imagery and empirically evaluated the effectiveness of various motion estimation algorithms for down-stream tasks such as action recognition from video sequences.
Intelligent systems not only require the relative motion of objects around them, but also a precise global location with respect to a map, i.e., for planning or navigation tasks. With LOST, we have demonstrated that localization solely based on map information is feasible. In Semantic Visual Localization, we further showed that semantic and geometric information can significantly improve visual localization, allowing for localizing wrt. the opposite driving direction or in the presence of strong environmental changes.
Moreover, we have developed Conditional Affordance Learning, a novel model for sensori-motor control which learns driving affordances from video sequences using only very limited supervision. To foster new research on 3D scenes in motion, we have created KITTI-360, a new dataset for 3D urban scene understanding, annotated at the object level.