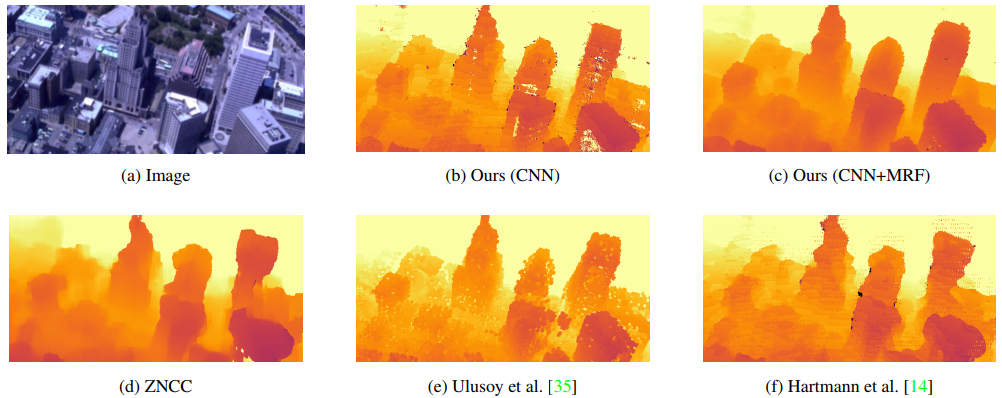
(a) An example input image to our algorithm (b-f) Comparisons of the estimated depth map using RayNet with state-of-the-art probabilistic and trainable methods as well as with two commonly used disparity methods. RayNet (CNN+MRF) consistently results in significantly smoother reconstructions while retaining sharp object boundaries.
Convolutional networks working on images are often agnostic of the image formation process such as perspective geometry and occlusion; it is often only of marginal interest. However, classical approaches to 3D reconstruction benefit greatly from explicit knowledge about 3D geometry and light propagation, as we have also shown [ ][ ].
With RayNet [ ], we have presented the first approach that integrates this knowledge into a deep 3D reconstruction model by unrolling a high-order CRF as layers in a convolutional network, combining the strengths of both frameworks.
Furthermore, knowledge about the types of objects being reconstructed constrains the possible set of outcomes. For this reason, we have leveraged the combination of semantic classification in the context of 3D reconstruction. In an initial phase, we have shown the benefit for classical techniques [ ]. In the same spirit as RayNet, we have subsequently leveraged the power of deep learning to perform the semantic 3D reconstruction jointly [ ].
The code that accompanies our CVPR 2018 paper can be found here. The dedicated documentation page can be found in our documentation site.
Towards Probabilistic Volumetric Reconstruction using Ray Potentials
This work presents a novel probabilistic foundation for volumetric 3-d reconstruction. We formulate the problem as inference in a Markov random field, which accurately captures the dependencies between the occupancy and appearance of each voxel, given all input images. Our main contribution is an approximate highly parallelized discrete-continuous inference algorithm to compute the marginal distributions of each voxel's occupancy and appearance. In contrast to the MAP solution, marginals encode the underlying uncertainty and ambiguity in the reconstruction. Moreover, the proposed algorithm allows for a Bayes optimal prediction with respect to a natural reconstruction loss. We compare our method to two state-of-the-art volumetric reconstruction algorithms on three challenging aerial datasets with LIDAR ground truth. Our experiments demonstrate that the proposed algorithm compares favorably in terms of reconstruction accuracy and the ability to expose reconstruction uncertainty.