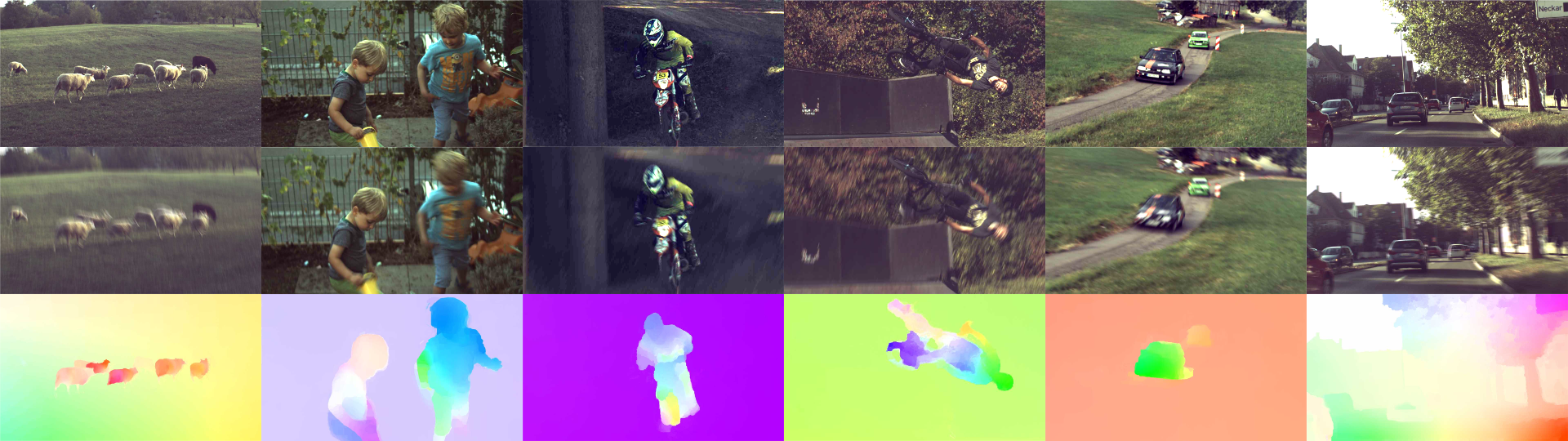
The upper row shows challenging scenes recorded with the high speed camera while the middle and lower row show realistic motion blur and reference data created with our approach.
Much of the recent progress in computer vision has been driven by high-capacity models trained on very large annotated datasets. Examples for such datasets include ImageNet for image classification, MS COCO for object localization or Cityscapes for semantic segmentation. Unfortunately, annotating large datasets at the pixel-level is very costly and some tasks like optical flow or 3D reconstruction do not even admit the collection of manual annotations. As a consequence, less training data is available for these problems, preventing progress in learning-based methods. As there exists no sensor that directly captures optical flow ground truth, the number of labeled images provided by existing real world datasets like Middlebury or KITTI is limited. We believe that having access to a large and realistic database will be key for progress in learning high-capacity flow models.
Motivated by these observations, we exploit the power of high-speed video cameras for creating accurate optical flow reference data in a variety of natural scenes. In specific, we propose an approach to track pixels through densely sampled space-time volumes. Our model exploits the linearity of small motions and reasons about occlusions from multiple frames. Using our technique, we are able to establish accurate reference flow fields outside the laboratory in natural environments. Besides, we show how our predictions can be used to augment the input images with realistic motion blur. We demonstrate the quality of the produced flow fields on synthetic and real-world datasets. Finally, we collect a novel challenging optical flow dataset by applying our technique on data from a high-speed camera and analyze the performance of the state-of-the-art in optical flow under various levels of motion blur.
We provide
- Supplementary Material
- Code and Documentation
- Benchmark Dataset
- Teaser High Speed Sequence
The complete high speed dataset will be following soon.