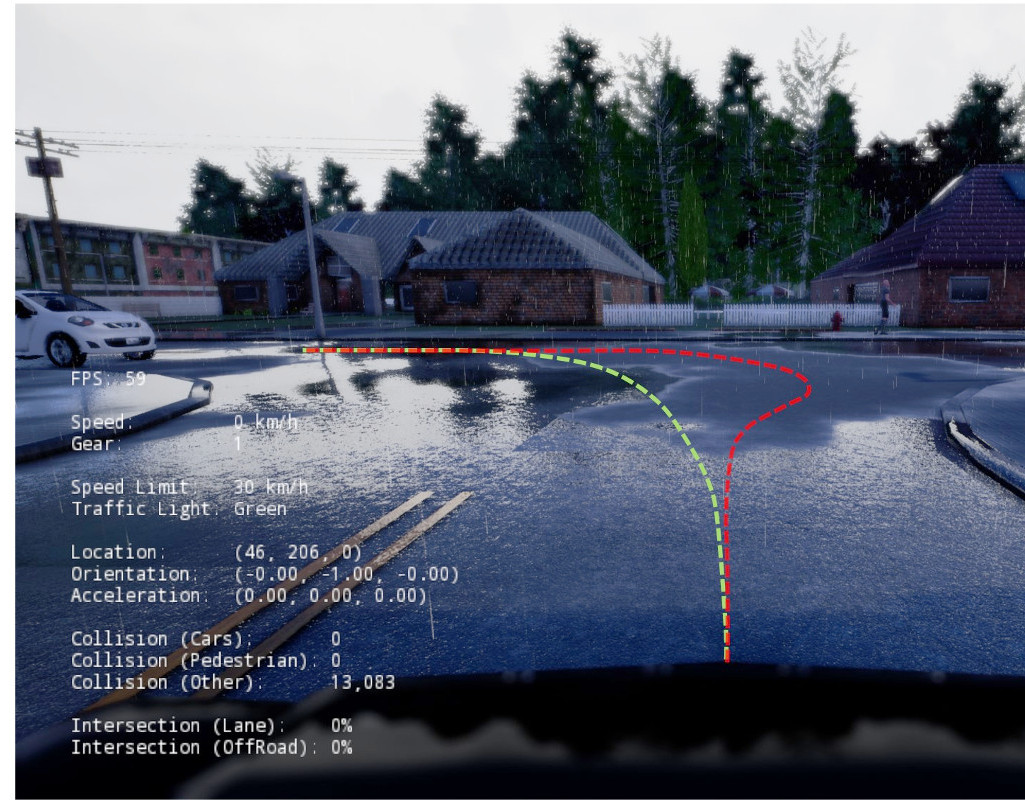
Intelligent systems not only require the relative motion of objects around them [ ][ ], but typically also a precise global location [ ] with respect to a map, i.e. for planning or navigation tasks. With LOST [ ], we have demonstrated that localization solely based on map information is feasible. For the creation of such maps, we have developed a method that correctly handles large-scale dynamic environments [ ]
In [ ], we further showed that semantic and geometric information can significantly improve visual localization, allowing for localizing wrt. the opposite driving direction or in the presence of strong environmental changes.
Moreover, we have developed a novel model for sensori-motor control [ ] which learns driving affordances (such as the presence of obstacles or red lights, or the current speed limit) from video sequences using only very limited supervision. To foster new research on 3D scenes in motion, we have created a new dataset for 3D urban scene understanding, annotated at the object level [ ].
To provide an overview on the current problems, datasets and state-of-the-art, we have written a review paper on the topic [ ].
Project page "Computer Vision for Autonomous Vehicles: Problems, Datasets and State-of-the-Art"
Project page "Semantic Instance Annotation by 3D to 2D Label Transfer"
Video on "Conditional Affordance Learning for Driving in Urban Environments"
Project page "Robust Dense Mapping for Large-Scale Dynamic Environments"
Video on "Robust Dense Mapping for Large-Scale Dynamic Environments"